Meet the Model That's Redefining Value and Plotting the Demise of Messi and Ronaldo
Say hello to G+
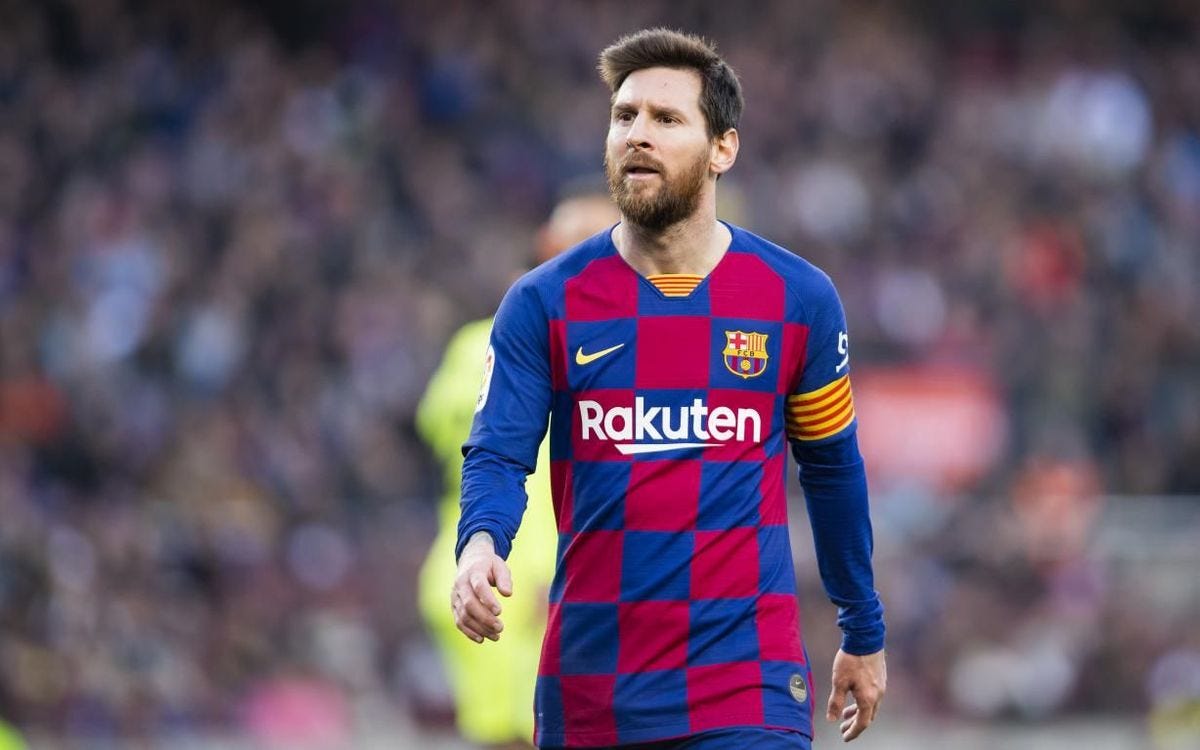
A couple years ago, I was talking with someone who ran the data side of a Champions League team that had recently made a run all the way to the semifinals. When I asked him to describe soccer’s current state of quantified things, he likened it to if basketball teams were only able to count dunks. Dunks are incredibly valuable, and the players who are constantly dunking are constantly producing high-probability scoring attempts. The current NBA dunk leader is also the defending MVP and the front-runner for this season’s award: Giannis Antetokounmpo of the Milwaukee Bucks. Next on the list is John Collins of the Atlanta Hawks, followed by Dwight Howard of the Los Angeles Lakers -- two solid contributors, in large part due to their ability to get the ball and shove it directly through the rim, but nowhere close to the second- and third-best players in the league. Except, if “dunks” were our only datapoint, then we might think that they actually were.
There’s so much more than dunking -- and there’s so much more than scoring or assisting. In soccer, stats like expected goals have given us a better understanding of how top goal-scorers score most of their goals -- constantly getting into good positions, rather than finishing their chances at an especially high clip -- and what players are most likely to keep scoring goals or stop scoring goals. Take a step back in the chain, and you’ve got expected assists: what players are playing the passes that lead to those high-quality chances. Given the low-scoring nature of the sport, players who do either of these things are providing something valuable, but it’s still only a small percentage of everything that goes on across a 90-minute match. What if there’s a player who takes great shots but constantly turns the ball over, or a guy who conserves all of his energy, rarely shoots, doesn’t defend, and focuses only on playing the killer pass?
To answer these questions, the folks at American Soccer Analysis created a metric called goals added, or “g+”. It’s the latest in a string of possession-value models that aim to put a value on everything that happens with the ball across a match. The ASA model is the slickest one I’ve seen yet in the public domain, and it works from a pretty intuitive premise: every on-ball action both affects a team’s likelihood of scoring and conceding a goal. They then ascribe the changes to those likelihoods to individual players, and eventually the model spits out, you guessed it, the number of goals that everyone has added. (A couple of the creators had a great chat with Mike Goodman on the Double Pivot podcast about the development and details behind the stat.) ASA’s work mainly concerns Major League Soccer, but they’ve also applied the G+ model to the European game. I talked with ASA’s John Muller about the creation of the model, what G+ teaches us about the sport, and why Lionel Messi might not be the best anymore.
Why did you guys create G+? And if you're explaining this to a soccer fan who's not necessarily familiar with analytics, how would you describe the stat?
American Soccer Analysis was originally a group of baseball sabermetrics nerds, and the guy who designs most of ASA's models, Matthias Kullowatz, has dreamed for years of creating a Wins Above Replacement-type stat for soccer. Goals added started as an attempt to answer the question, "How much is any given player contributing to his team's success?"
That's a way harder question to answer in soccer than in baseball, but the basic framework of goals added is pretty intuitive. To start off, we value any situation on the pitch by asking a machine learning algorithm two questions: what's the chance that the team with the ball will score on this possession, and if they turn it over what's the chance that the opponent will score on the next possession? The two-possession horizon reflects the fluid nature of soccer and means we're really measuring something more like probable goal difference added, which is, you know, how you outscore opponents and win games.
But so then once we've valued the possession like that, anything that happens on the ball can be measured in the same goal units, according to how much it changes the two-possession scoring probabilities. A progressive pass will make it more likely that your team will score and less likely that the opponent will. A successful tackle will make it less likely that your opponent will score and more likely that you will. The cool thing is that we're no longer just counting up passes and tackles and then making a judgment call about which per-90-minute volume stats we want to put on a radar because we think they're probably useful. We've got a single metric that allows us to compare and combine all of these on-ball contributions and see how much they actually matter to the scoreline.
I imagine when you're undertaking something like this, you're looking for it to both confirm some ideas we hold about the sport while also creating some new knowledge. Do you have any examples of things that the data confirmed and new things it taught you about how soccer works?
One problem that possession value models like g+ have confronted is the question of how to value strikers. If you award all the credit for each pass to the passer, as most folks instinctively do, then you're kind of stuck defending the position that all the guy in the box brings to the table is the ability to turn a pass into a shot by kicking it in the direction of the goal. Which, look, my soccer career topped out in a 11-year-old suburban rec league and I can do that. Now, you could choose to give the shooter credit for increasing his team's chance of scoring to 100% on possessions where he scores and reducing it to 0% when a shot misses or is saved, but those values are going to be super volatile because they reflect the mostly random noise that fans new to soccer stats mistake for "finishing skill." That's not where strikers add the bulk of their value either. What makes good strikers good is their smart movement and physical off-ball work to receive a pass in a dangerous shooting position in the first place.
One of my favorite features of goals added is that it splits pass values between the passer and receiver, while mostly minimizing shot value. And it turns out when you do that, suddenly all the really good strikers rise to the top and you start to see a better fit between g+ and compensation, because now the model is more accurately capturing forwards' skillset. It's kind of a sneaky way to value at least some off-ball contributions using event data, which is bad for obvious reasons at recognizing off-ball contributions. Receiving value is a choice that we imposed on the model, but the results speak for themselves. That's a way this project has helped confirm something I thought I knew about soccer.
Something the model's made me think a lot about is the relative worth of possession and field position. As a Barcelona fan, I like to watch teams that dominate the ball, and I think that because nearly all elite teams in the last decade have been ball-dominant, a lot of smart soccer people have spent a lot of time talking about the value of possession while pooh-poohing primitive old Route One tactics. Goals added complicates that picture. The model thinks a lot of possessions are worthless, in the sense that regardless of which team is nominally controlling the ball, both sides have about the same chance of scoring over the next two possessions. I've looked at one play where a guy in a neutral situation like that had the bright idea to try to chip the opposing keeper from his own half with a ridiculous 80-yard lob. Surprise, surprise, it didn't work and he looked dumb. But the model decided that the shooter had actually improved his team's situation, because now the keeper had the ball in his own box and his team was less likely to score than to turn it over in a dangerous position. The dumb shot was functionally equivalent to bringing on the punting unit at the 50-yard line and downing the ball at the one.
Is that right? Should everyone be trying 80-yard shots? I'm going to go out on a limb and say “no”. Sometimes with models it's just weird shit in, weird shit out, you know? But I think the model is pointing to something that the smart soccer people have figured out about possession, which is that the whole disorganizing-the-opponent-with-passing-patterns thing is sort of a sideshow, and the main reason elite teams are elite is that they get the ball into the opposing half and keep it there. Guardiola's Barcelona may have been fun to watch because of their passing but they were great because of their counterpressing, as Klopp's less intricate but equally lethal teams have shown us. Possessions that start high up the field are more likely to score. Doesn't matter how you play or how good you are, that's just a universal truth.
Actually, the team I thought of right away on the field position thing was Jesse Marsch's 2018 New York Red Bulls, who were sort of the apotheosis of the all-pressing-no-possessing style. Booting away worthless midfield possession in order to press high was their jam, and they were phenomenally successful at it, at least in the simplistic world of MLS. But then guess what, just this morning Marsch won a European league trophy with another Red Bull team by doing pretty much the same thing. Maybe we're leaving the possession decade behind and entering a new field position era. I'd hate that, personally, but the model probably wouldn't.
One of the things you always hear from data folks -- and I believe you've mentioned this -- is that when you're creating a player-based metric, you'll know you fucked up if Lionel Messi isn't at or near the top. How does Messi rate out by G+? And does this data tell us anything new about what makes him so good?
The old Bill James chestnut is that if four out of five times a new metric tells you what you already know, but one out of five times it surprises you, you might have something worthwhile. The unofficial soccer corollary to that is that Messi had better be one of the four. It's convenient that the GOAT happens to play at a time when we're developing all these increasingly complicated new stats, because it's an instant check on your stat's credibility.
I'm pleased to announce that goals added passes the Messi test. That wasn't a given. Bear in mind that this model doesn't care about actual goals at all, so just scoring a shit ton of them doesn't guarantee that it'll love you. In fact, since Messi is one of the rare players whose finishing consistently destroys xG models, there was a chance that g+ might underrate him. Thankfully he contributes so much in so many ways that his career goals added rate over the last seven seasons is the best in Europe by a wide margin. Five of the six best individual seasons across top leagues in that span belong to Messi. In other words, the model is telling us what we already know.
What I love is that the model shows the shifting ways he's created that value. For analysis purposes, we divide goals added into six buckets: shooting, receiving, passing, dribbling, interrupting, and fouling. In 2012-13, Messi's most valuable category was dribbling (man, I miss dribbling Messi). During the MSN years he created about half his g+ from passing, but with so much help around him he was also adding a ton of value from receiving and shooting. After Neymar and Iniesta left and Messi had to shoulder most of the creative load, you see his receiving drop—he's not getting fed in the box as much anymore—and his dribbling bounce back a little, while his passing stays super high. There's been a lot written on Messi's different phases, but it's cool to see them defined so clearly by this all-in-one metric.
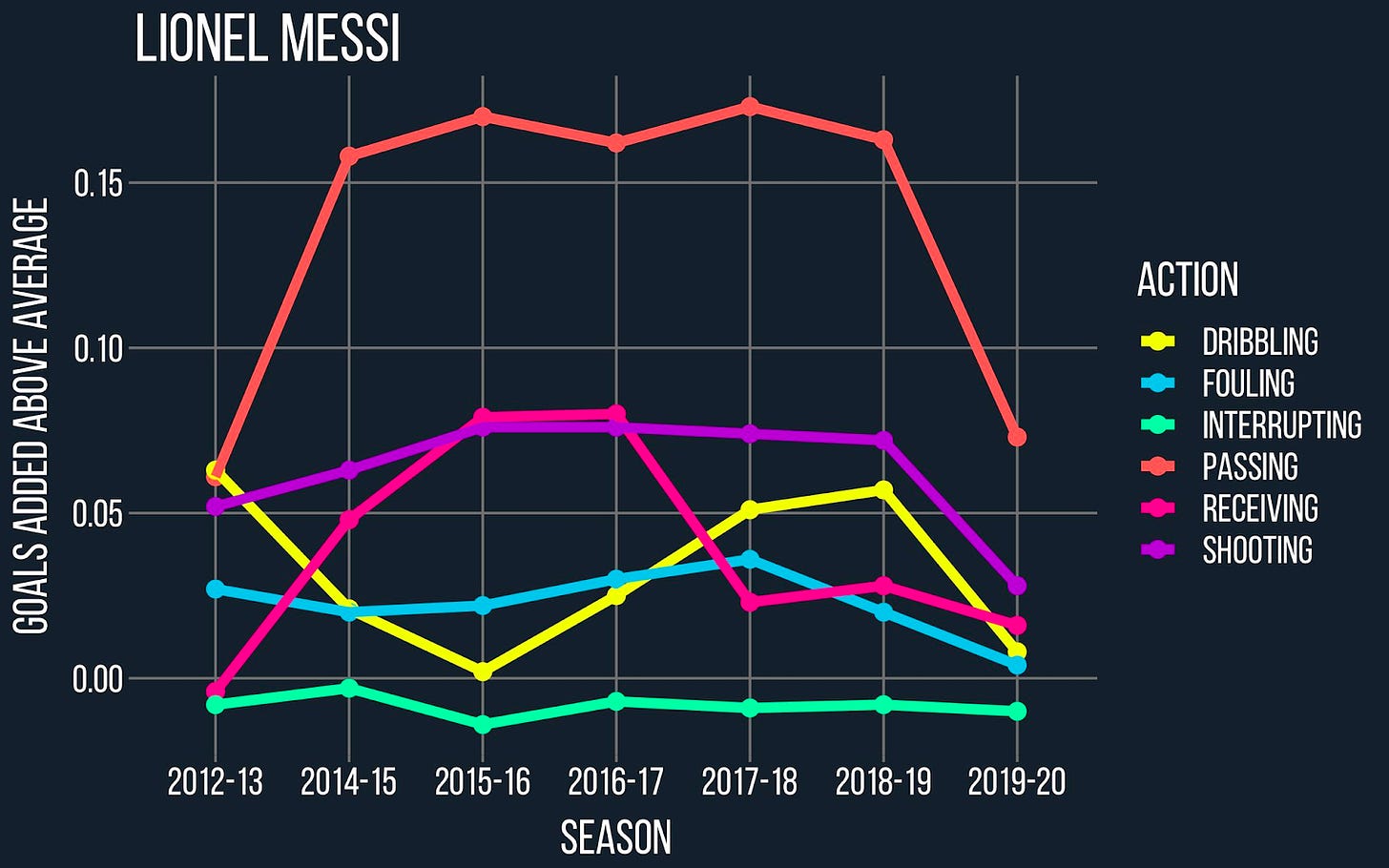
By way of contrast, the model loves Cristiano Ronaldo, too, and even thinks he added more value than Messi in 2012-13 (which happens to be the year he reclaimed the Ballon D'Or), but his goals added has always come overwhelmingly from receiving—he's incredible at getting on the end of passes into the box. One thing we've noticed is that receiving values generally show a more distinctive age curve than the other buckets, and sure enough Cristiano's seen a steady decline in his receiving g+ in his thirties without reinventing himself as a creator like Messi has.
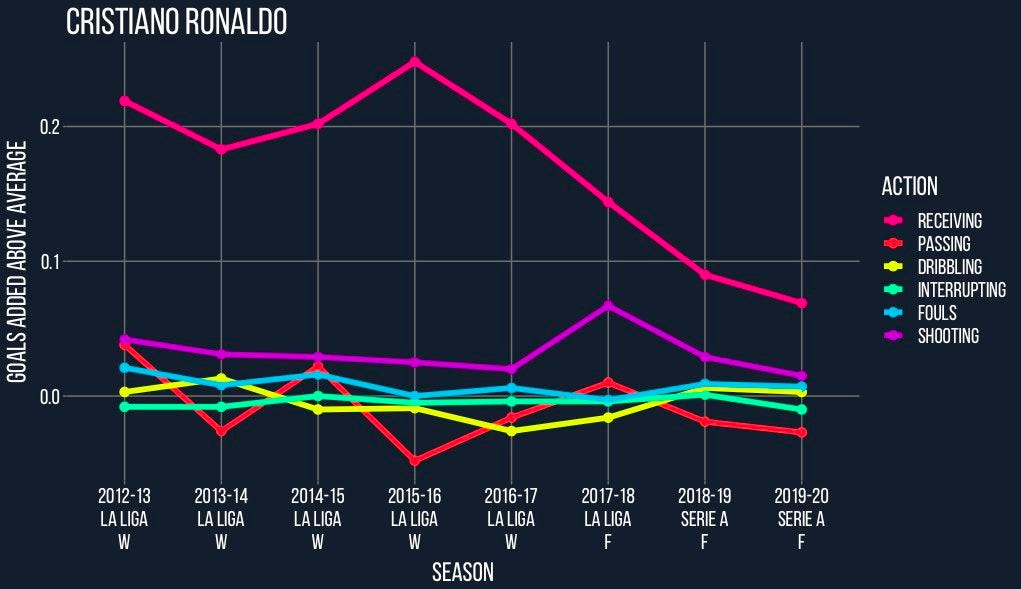
Messi's numbers this year look as good as ever. In fact, his non-penalty goals+assists/90 rate (1.33) is essentially exactly in line with his career average of 1.32. But you guys see a relatively steep decline in his performance this season. What's changed? I've always kind of looked at the future of soccer analytics as finding ways to value all of the players who aren't scoring and creating, but it seems like, at least in this particular case, your work is showing that goals+assists can actually obscure how valuable a player has actually been. Is that right?
That's a great point. A glance at Messi's conventional stats, even stuff like non-penalty expected goals plus expected assists per 90 minutes, doesn't suggest anything like the sharp decline that we've seen in his goals added this season. He's gone from consistently producing at least +0.30 goals added per 96 minutes more than the average player at his position, which is off-the-charts nuts, to just +0.10 g+avg this year, which is, you know, still like the 98th percentile. His numbers have fallen in every g+ bucket, especially passing. Over on the more familiar stats tables, his non-penalty xG is down something like 15 percent (with Suárez hurt and Antoine Griezmann a bad fit, Barcelona's had trouble getting Messi the ball in the box) but his xA looks totally normal. So why does his passing g+ suddenly suck?
This is speculative, but I'd guess one major thing that's dropped off is his incredible pass-before-the-key-pass playmaking. Young false nine Messi used to slide the ball through to a winger and then magically appear unmarked at the penalty spot to slot home the return pass; for the last fiveish years his trademark play has been those pinpoint curling balls over the entire defense onto an overlapping Jordi Alba's foot. You won't get any xA for either of those passes, since they don't directly set up a shot, but you'll get a ton of g+ because the model can recognize how much more threatening they make the possession. This season Alba was out injured for a good chunk of the fall and never really got back up to speed before the pandemic hit. Griezmann and Ansu Fati haven't provided the same kind of wide target for diagonals on the wing. So while Messi may still be setting up dangerous shots with passes directly into the box, he's doing less playmaking further back in the chain. You can see some of that in his shot-creating actions on FBRef, which are down 18 percent since last season, but goals added may be even more sensitive to the problem since it can evaluate Messi's actions whether or not they lead to a shot.
What's next for G+ -- both in terms of what you want to dig into using the framework you've created and in terms of what you want to add to it?
What's next is using goals added for actual game analysis now that soccer's coming back. The ASA crew has spent a lot of time trying to get the first draft of the model right but is just getting started thinking of creative ways to use this new tool to learn stuff about soccer beyond, like, "Messi good." If you're curious to see where this project goes, follow @analysisevolved on Twitter, because you'll definitely see a lot of new work with g+ over the next few months.
As for what I'd like to add to g+, I've been pretty vocal about hoping to fix the way the model handles defense. Right now players are credited for defensive actions according to the value of the possession that they broke up. There's a certain logic to that—the most valuable interrupting action under the current framework would probably be a goalline clearance, which is undeniably a good thing to do—but I think it gets defense backwards. The most valuable interrupting actions, the ones you'd most like to see your players doing, are the ones that happen high up the pitch, where opponent possessions aren't very valuable at all. Field position and all that. What I'm pushing for is some kind of zonal system where we use a player's touches to infer his area of defensive responsibility, then debit him for a share of opponent goals added generated in that zone. That seems conceptually sounder, but some of the smartest analysts working have tried similar things over the years and that first inference step turns out to be really hard. It'll be fun to see if ASA can crack it.
If you enjoyed this, please subscribe. And please pass on the word to anyone you know who might be interested. Call your boyfriend. Tell your girlfriend. Inform your mortal enemy. Everyone is welcome … unless you’re a fascist — in which case, get the hell outta here! Also, please consider donating to these community bail funds. Thanks, as always, to all you non-fascists for reading along.
The defensive roles are counterintuitive in some ways. ‘Showing him outside’, or ‘onto his weaker foot’ is the positional forcing of a weaker choice on the opponent. Only the very best offensive players are equally effective on either side/foot for example, but the best defenders will still usually force a backward or poor choice by the attacking players, even if they don’t intercept, block a shot or tackle. Positional awareness is at a premium. The relationship between defensive players when defending -in pairs & triangles, and in complementary attributes - matters greatly. Comparing attacking & defending is almost like magnetic field opposites, of attraction and repulsion
I would love to see what this model has to say about controversial geniuses like Özil and what their performance has been like in recent seasons